Options trading as a widget factory
Edge Functions: the hurdle for a trader's unit economics
This is part 2 to When it's normal to have no idea what your returns are
Traders don't think about rates of return explicitly in the same way traditional investors or fund managers might. A trading firm is more like a holding company where each portfolio business is autonomous, while the mothership scales risk management and costs of shared resources, especially technology.
The parent company is the master allocator weighing not just opex decisions but how much capital to devote to each strategy. The capital is both the cash in the margin and some invisible cushion. From the individual trader’s point of view that cushion is an explicit or implicit risk budget — “how much can my p/l swing without inviting management to my inbox, or worse, my desk?”
Management’s thinking will be guided by ROI and hurdles. High-level stuff that overlaps with “being an investor”. The traders will have a process-oriented business lens concerning themselves with the unit economics of their specific form of artisanal bookmaking. The traders’ focus makes sense. It’s keeping their eye on the ball, understanding the role they play, why it pays, and how the information they glean from each day’s match feeds back into the gameplan.
Mechanically, unit economics, ie dollars at the trade level, is a natural focus for a trader in this structure. The master’s allocation to the trader can flex with the opportunity. That’s an advantage of the holding company or pod structure. The trader isn’t strait-jacketed into a strict AUM that leads to cash drag in dull times and wasted opportunity when it’s sizzling. But the “floating” AUM means the denominator in a return calculation is a moving target.
With this in mind, we can think about the answer to the following question from the perspective of what his counterparty, the market-maker, considers when they provide liquidity.
How important is (il)liquidity in options when making risk-defined trades such as credit/debit spreads or buying single call/put options?
The widget factory
Scenario
You’re a professional options trader. In coming up with the year’s business plan, you estimate you can trade 100k option lots per day netting a penny of edge per contract.
Your annual expectancy is:
100,000 contract x $.01 x $100 multiplier x 250 trading days = $25mm
Call it $2mm per month. Maybe you take a few weeks off and the desk is less productive when you’re out if you want to flatter yourself.
Annual expectancy is $24mm.
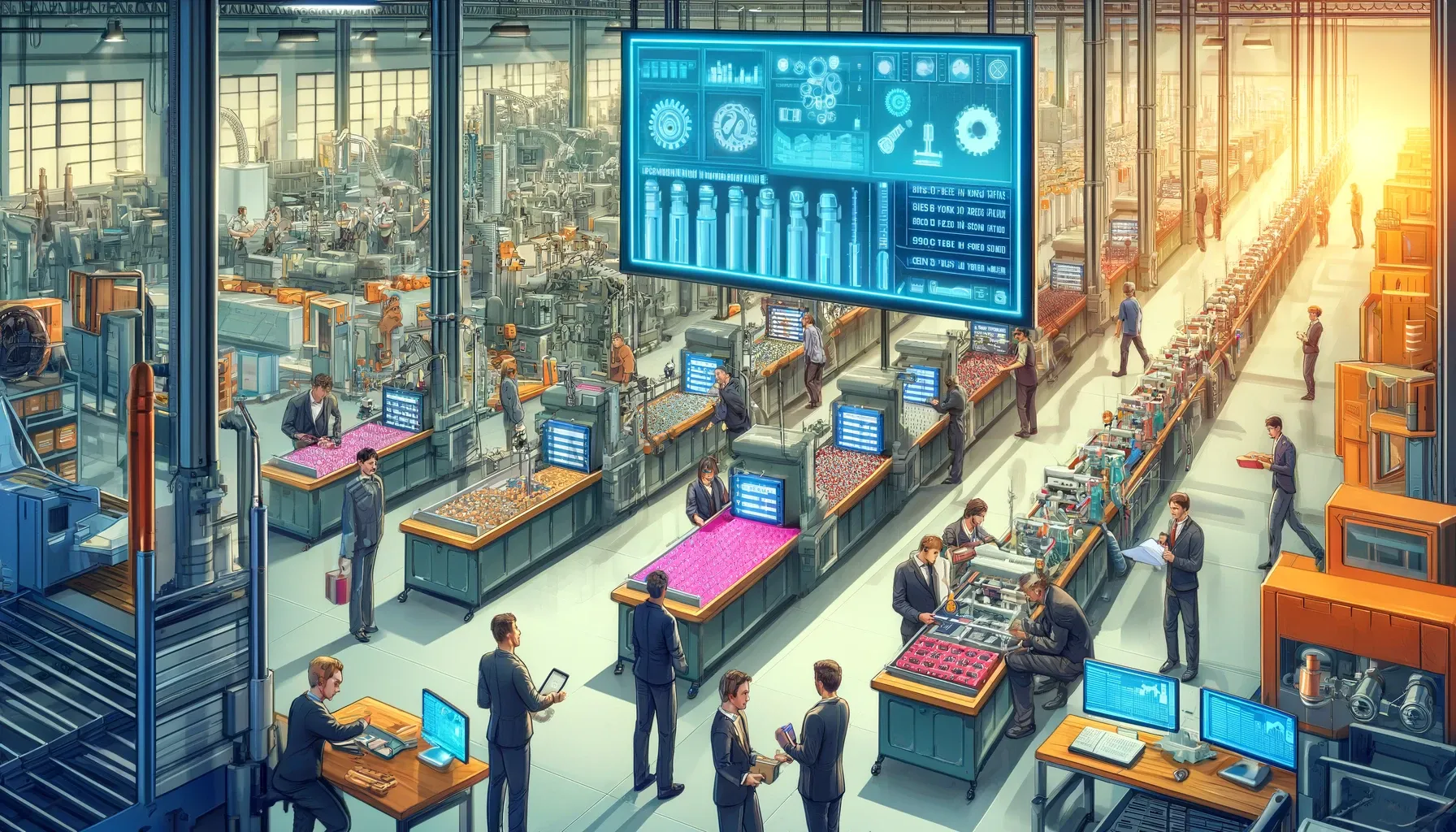
You also believe you can do this with a monthly standard deviation of $5mm.
Your monthly Sharpe is 2/5 or .40
Your annual Sharpe is 24/5√12 or 1.4
You, and more importantly, management believe this can be done with margin of $100mm give or take $25mm in a typical year. This is deemed an appropriate use of capital after considering the costs of paying the traders who contributed.
Zooming in on a single day
We will continue with the implicit assumption that edge stacks linearly and volatility by root time.
If there are 21 trading days in a month, you expect:
daily p/l = $2mm/21 = $95k
daily p/l volatility = $5mm/√21 = $1.09mm
This gives you a sense of how much p/l and its variation should accumulate over time. The amount of edge and risk you take should pile up to get you in this ballpark.
If your p/l vol is much smaller or larger it can help you answer diagnose questions like:
- Am I delta hedging too little/too much?
- Should I be trading bigger/smaller and if I change my size do the performance metrics scale as predicted?
But this is also a way to develop a sense of proportion about the edge per trade.
Imagine the following call option (assume 0% interest rate)
- Stock = $20
- Strike price = $22
- Days to Expiry = 30
- Fair Implied Vol = 47%
- Fair premium = $.40
- Call delta = .26
- Option vega (sensitivity to a 1 vol point move) =$.019
A fund wants to sell 1,000 of these to overwrite a long stock position and wants to know your bid.
How do you price it?
First, because it’s a stock overwriter you don’t suspect adverse selection. It’s a natural order. The screen might be showing a $.30 bid for the option and the broker showing you the offer expects you to show a better price if you want to keep seeing flow. In addition if you show too little improvement from the screen bid, the broker may feel he can get a better price from a competing trader.
You need to balance:
- your own desire to get the lowest price
- the broker’s willingness to shop the offer elsewhere
- how much edge you need to compensate you for the risk and costs associated with the position
#1 and #2 are game theory questions. Quants will have a model. A trader picking up the phone has experience to lean on — they know how competitive this particular name is, the broker’s tendencies, at what price the trade can be “crossed” on an exchange without awakening price improvement, and other involuntary muscle movements.
For this discussion, we are concerned with #3 since it represent the minimum edge you require to do the trade. In this context it establishes your maximum bid and is therefore the upper constraint on your bid while #1 and #2 put a floor on your bid.
Defining an edge function
We need starting assumptions about the stock’s liquidity. I’ll make one up but you should know that is a large area of quantitative research.
If you buy 1,000 .26 delta calls, you need to sell 2,600 shares of stock to hedge. We’ll assume the stock is thin and the market impact of 2,600 shares is a dime.
Compensation for slippage = delta x slippage
Compensation for slippage = .26 x $.10 = $.026
So just to compensate us for delta impact, we require 2.6 cents of edge. Shaving that off fair value of $.40 leaves the buyer with a slippage-adjusted fair value of $.374
Delta is a relatively straightforward risk.
The primary risk we care about is vega or vol risk. This is what we are in the business of actually trading.
If implied vol drops from 47% these calls will lose value. Suppose IV in the name is whippy and the daily standard deviation of strike vols is 5 points. With a vega of $.019, these options would lose a dime of value if IV dropped 5 clicks.
Given the vol of vol, you decide you need 1 full vol point of edge to warehouse the option. Your slippage-aware price of $.374 is deducted against for the edge you require…your maximum bid is $.374 - $.019 = $.355
Then there are per contract exchange and commission fees. This is a labyrinth that depends on what type of entity you are (market maker, customer, pro customer), which of the 17 exchanges the trade prints on, whether the trade is voice or electronic, option class, and whether you are taking or adding liquidity. Here’s a look at just the CBOE’s fee schedule. Exchange competition also means changing fee schedules.
We will simply round the fees + commissions on our hypothetical voice trade to a penny.
Therefore our maximum bid starts with a fair value of $.40 and deducts
-$.026 for delta slippage
-$.01 fees/commissions
-$.019 compensation for taking vega risk (ie the reason for our business in the first place)
Our maximum bid = $.345
[And yes, options trade in half cents in the voice market.
“I’m thirty four and a half cent bid, no bill”…IYKYK]
This is a stylized edge function but it’s quite intuitive. Lower vol, lower vol of vol, more liquid names will have tighter option markets. The option market inherits liquidity from the underlying market.
[This isn’t always true. There are periods of intense option trading competition where banks or market makers are trying to capture market share in a slow market. This leads to situations where the options do not price enough delta slippage — or as we say “the options are providing more liquidity than the underlying” offering smart directional traders an opportunity pick off deltas.]
Additional real-life points:
- Options have interest rate risks. Rho is the greek known as sensitivity to interest rates. But bonds are liquid so rate risk is easy to hedge. If you are getting too offsides on rho risk you likely have a bad interest rate feeding your option model causing you to be a structurally high bid in calls and low offer in puts (assuming your running too high of an interest rate). This is not a common concern.
- If a name is hard-to-borrow, shares are scarce and the rate to borrow can fluctuate wildly. This will reflect itself in wider option markets since cost of carry is a major input. In this case, the edge function is likely to explicitly incorporate rate risk.
- Real life edge functions are position-ware. If this customer keeps selling me calls, I’m going to require more edge to add to my position. The edge functions behind streaming quotes reflect market-maker leans to buy or sell deltas and vegas. For example, if I’m long XYZ deltas, rather than cross the bid-ask spread in the stock I might offer calls and bid puts very close to fair value to let contra flow reduce my delta risk naturally. And I might even get paid a rebate if I’m posting.
- Highly liquid markets with lots of native order flow are less reliant on market makers. SPY options have loads of natural buyers and sellers and will trade tighter than any market-maker’s edge functions would recommend.
- Option spreads can offset greeks. But it’s ambiguous. Referring back to our example. If the customer tried selling the 22-24 call spread instead of the 22 call, the vega would have only been $.009 instead of $.019 and the delta would have been .16 instead of .26. This means less delta slippage and we could likely pay a full penny more with the reduction in vol risk.
But there’s a big gotcha…
Commission and exchange fees are on a per contract basis so the fixed costs of trading a spread is twice the cost of trading a single option. And if you trade more spreads because the risk is lower there might be no relief in pricing that you’d expect for trading a less risky structure! - Intelligent, scaled, efficient trading shops who can see lots of flow have more outs for their risks. They can internalize deltas against more contra flow in other parts of their business allowing them to hedge both deltas and vol risk less often. This allows them to price even tighter (ie more aggressive edge functions), which leads to more happy brokers and seeing more flow. It’s a flywheel. The Matthew effects are huge in trading. A worthy callback to this idea is post from the archive: You Don’t See The Whole Picture
In sum, edge functions tie the unit economics of individual trades back to the broader business metrics by modulating how much compensation for risk one requires.
The cost of liquidity depends on risk (dynamic) and fees (more constant) which are passed along to end users through edge functions.
The goal was to overdeliver a response to the reader question:
How important is (il)liquidity in options when making risk-defined trades such as credit/debit spreads or buying single call/put options?
Hope it was helpful!