Mr. My Way
Sports gambling and a book rec
First I want to bring an enlightening podcast episode to your attention.
🎙️How a Professional Sports Bettor Really Makes Money (Bloomberg Odd Lots)
Joe and Tracy interview pro gambler Isaac Rose-Berman. In under an hour, you can learn a ton about the sports gambling industry. With sports leagues shoving it down our throats, young and old alike, I feel it’s important to understand what’s going on. And it’s not pretty.
I’ve talked about how this industry is rigged in “Free” Markets Wet Dream. This podcast echoes the problems but it encompasses so much more — opportunities, cultural impact, and basic mechanics. There are lots of misconceptions about sports gambling as well.
This is a list of some of the less-obvious ideas found in the interview:
Professional Sports Betting Strategies and Challenges
- Identifying Market Inefficiencies: Spotting mispriced odds and exploiting gaps in bookmakers' knowledge, especially in niche markets.
This exchange is the betting parallel of fundamental vs arbitrage investing:
Tracy (07:49):So just so we can better understand this dynamic, walk us through your sort of day-to-day as a professional sports gambler. What kind of opportunities are you trying to identify and then how do you decide how much money, for instance, to apply to each individual bet?
Isaac (08:05):Yeah, so it's a great question. So it really depends on what sports are in season. So right now, you know, end of the NBA, but there's a lot of MLB things like tennis are year round. And so it does depend on the sports.
There are in general two ways of identifying profitable sports bets. The first is you can take sort of a market-based approach. And by a market-based approach, what we mean is there are tons of sports books all out there and as Joe mentioned, they're all offering all of these different kinds of bets. And if you constantly scroll through all of the odds, you're going to find slight mispricings. So let's say everybody has the Yankees as two-to-one underdogs and one sportsbook has them as a three-to-one underdog. You can identify that as off market and you can place that.
Tracy (08:45):Oh, I see. So you're not saying that the platforms have the odds wrong, you're trying to identify outliers among the platforms.
Isaac (08:51):That's exactly right. yeah. So that's probably the main that the majority of professional or winning sports betters make money is by identifying markets which are simply mispriced. And for that you don't need any special sports knowledge, you just need to have a screen with all the odds and constantly be scrolling through them and looking for price changes and looking for books that are slow to update.
The other way to do it, which is a lot harder and a lot [more] rare, is to basically create your own numbers. So you say, okay, I know exactly how much each player is worth. I know what the weather is going to be today, I know these matchups and so I'm going to generate kind of from scratch from my own, model the odds and then apply that to the market. And when it comes to major liquid markets like the NFL or the NBA or the MLB, that's really, really hard to do. And there are very, very few people who can do that, but those are the people who make the most money. - “Flipping Whales”: Partnering with high-rolling bettors who are often losing money but have high betting limits, allowing professionals to place larger bets indirectly.
- Account Limitations and Closures: Sportsbooks limit or close accounts of consistently winning players. In fact, the first few bets you make on a platform are given a heavy weight in determining your betting limits. If you open an account to arb a line, the platform knows it because they know what the sharp side is. To get a healthy bet size limit you want to open an account with trades that casual amateurs make. Bet on the home team. Go for some parlays. Be a sucker.
- Maintaining Multiple Accounts: To circumvent limitations, professional bettors often manage accounts across various platforms and use accounts of friends or family members.
Regulatory Concerns and Societal Impacts
- Match-Fixing Concerns:
- Lower-Profile Sports Vulnerability: More prevalent in sports where athletes earn less, making them susceptible to match-fixing offers.
- Risky Prop Bets: Bets on specific player performances increase the risk of manipulation.
- Monitoring and Enforcement: Challenges in maintaining integrity across global sports markets.
- Addiction Risks:
- Young Men: Disproportionately affected due to emotional attachments to sports teams and delusional beliefs about their betting acumen.
- Mobile Access: Easy access through apps exacerbates addiction potential, making gambling omnipresent and more enticing.
- Lack of Transparency:
- Tracking Wins/Losses: Many platforms make it difficult for users to track their overall performance.
- Deceptive Advertising: Emphasis on potential wins while downplaying the risks involved.
- Targeting Minors: Use of "social" sportsbooks and fantasy sports apps to attract younger audiences.
- US vs. European Models:
- European Practices: European sportsbooks are known for being less accommodating to winning players, often quickly limiting or banning them.
- US Adoption: Many US sportsbooks, owned or influenced by European companies, adopt similar practices, focusing on market share and profitability by restricting successful bettors.
Technological Aspects of Sports Betting
- Mobile Apps and Online Platforms:
- 24/7 Betting Access: Allows for instant betting from anywhere at any time.
- Variety of Bet Types: Including live in-game betting and exotic prop bets.
- Engagement Tactics: Use of push notifications and personalized offers to keep users betting.
- Data Analysis and Algorithmic Pricing:
- Initial Odds Setting: Advanced statistical models and real-time adjustments based on betting patterns.
- Third-Party Providers: Specialize in generating odds for niche markets, contributing to the breadth of available bets.
- Geolocation and Identity Verification:
- Regulatory Compliance: Ensures users are betting within legal jurisdictions.
- Preventing VPN Bypass: Ensures bettors cannot circumvent geographical restrictions.
- KYC Processes: Verifying age and identity to comply with legal requirements and prevent underage gambling.
Future Outlook and Potential Reforms
- Advertising Regulation:
- Restrictions on Ads: Calls for limits on the frequency and content of gambling advertisements.
- Honest Marketing: Need for ads that accurately depict the risks of gambling, including mandatory disclosures of odds and average losses.
- In-App Transparency Improvements:
- Display of Wins/Losses: Clearer, real-time tracking of a user’s total betting performance.
- Responsible Gambling Tools: More prominent tools and resources to help users manage their gambling.
- Research and Data Collection:
- Independent Studies: Need for unbiased, non-industry-funded research on gambling impacts.
- Tracking Problem Gambling: Better data on the prevalence and demographics of problem gambling.
- Regulatory Effectiveness: Evaluating the impact of different regulatory measures.
- Enhanced Responsible Gambling Tools:
- Opt-Out Limits: Consideration of default deposit and time limits that users must opt out of, rather than opt in to.
- AI Integration: Use of artificial intelligence to identify and address problematic betting patterns.
- Improved Self-Exclusion Programs: Streamlined processes for self-exclusion across multiple platforms to help problem gamblers limit their activity.
Economic Reality of Gambling Companies
- Misconception of Profitability:
- Despite the significant growth and visibility of the sports betting industry, many companies are not as profitable as assumed.
- Customer Acquisition Costs: Sportsbooks spend heavily on marketing, promotions, and sponsorships to attract new users. These costs often outweigh the revenue generated from bets.
- High Operational Expenses: Maintaining compliance with regulations, technology infrastructure, and customer support adds to the costs.
- Competitive Market: The need to offer competitive odds and bonuses to attract and retain customers further squeezes profit margins.
- Focus on Market Share: Many companies prioritize expanding their user base and market share over short-term profitability, leading to significant investments in customer acquisition and retention.
- iGaming as the Future:
- Strategic Shift: Many gambling companies see online casino games (iGaming) as their future primary revenue source. These games are more addictive and provide higher margins due to their rapid play nature and the continuous betting opportunities they offer.
- Regulatory Landscape: iGaming is currently legal in fewer states compared to sports betting, but where it is legal, it dominates revenue figures, indicating its profitability.
- Predatory Practices:
- Increasing Losers' Bet Limits: Sportsbooks often increase betting limits for users who consistently lose money, encouraging them to bet more and lose more. This contrasts sharply with the practice of limiting or banning successful bettors.
- VIP Programs: Offering special incentives and higher limits to big losers, which can exacerbate their gambling problems and financial losses.
The house edge on typical bets in sports is in the ballpark of 5% if you have have to risk $110 to win $100 on a coin flip. The house edge is ensures a healthy long term profit for the sportsbooks if they can avoid smart bettors. But the edge is small enough to keep bad bettors coming back. They win enough to think they have a chance.
On my flight back from NJ, I read applied mathematician David Sumpter’s The 10 Equations That Rule The World (he was the author of the sports analytics book Soccermatics as well).
It’s a good book for introduction to the below topics especially since it provides lots of real-world applications about problems we encounter on a regular basis. For example, he uses Bayes’ Theorem to show why forgiveness is not just a gracious thing to do but a statistically sound choice. He also bodies Jordan Peterson if you’re into that.
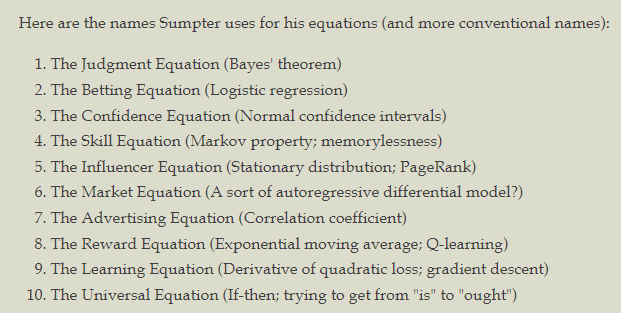
The book is sequential — the equations build on preceding ones to build rich models that underpin profitable business and life decisions.
Taking the baton from Odd Lots, we can use chapter 3’s Confidence Equation and chapter 6’s Market Equation to establish a basis for determining if we actually have an edge.
Let’s get into the details.
Chapter 3: The Confidence Equation
Sumpter defines the equation:
h * n ± 1.96 * σ * √n
where:
h = edge or signal per trial
n = trials
σ = standard deviation
The 1.96 gives away equation’s identity — it’s the 95% confidence interval.
The best way to understand it is by demonstration.
If you have 3% edge on a bet with a standard deviation of 71% and make 100 bets your realized edge will be:
.03 ± (1.96 * .71)/ √100
.03 ± .14
Your realized edge will have a 95% chance of falling between -11% and +17%
While the confidence interval contains zero, you cannot be particularly sure that the signal is positive and that the gambling strategy works.
The value of this equation is often best seen in reverse. We can invert the expression to ask:
“How many trials do I need to be 95% sure that my edge is positive?”
h/σ > 2/√n
*Note: The 2 comes from rounding 1.96 up. Sumpter doesn’t mind sacrificing precision to make the formula memorable.
💡Moontower readers will observe that h/σ is a measure of risk to reward and can be interpreted as a Sharpe ratio.
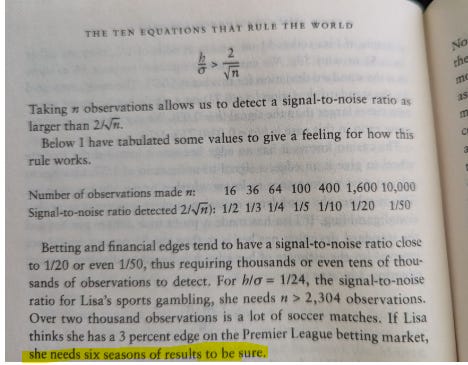
The bet described above is ascribed to a hypothetical gambler named Lisa. Notice that Lisa not out of the woods even if she gets to make this bet 2,300x.
Sumpter explains the problem:
During those six years, other gamblers might have picked up on her edge and started to back it. The bookmakers may adjust their odds and the edge disappears. The risk for Lisa is that she doesn't realize that her edge has gone. It takes over one thousand matches to be confident that an edge exists. It can take just as many expensive losses to realize that it has disappeared. The profits that grew exponentially fast now crash down and decay exponentially fast.
Notwithstanding the ever-present problem of “did the world change while I was deploying my strategy” the blunt math is instructive:
Most amateur investors are vaguely aware that they need to separate the signal from the noise, but very few of them understand the importance of the square root of n rule that arises from the confidence equation. For example, detecting a signal half as strong requires four times as many observations, and increasing the number of observations from 400 to 1,600 allows you to detect edges that are half as large. It is easy to underestimate the amount of data needed to find the tiny edges in the markets.
These ideas were fundamental in options training. You can see them applied in:
Understanding Edge (10 min read)
If You Make Money Every Day, You’re Not Maximizing (23 min read)
During the 1700s, mathematician de Moivre pioneered combinatorics (i.e., how many ways can you be dealt a full house). The combination formula relies on factorials which become computationally impossible when numbers get large, especially in the 18th century. Scottish academic James Stirling showed how, at large n, the binomial distribution can be approximated by the normal bell-curve.
In 1810, Laplace developed the idea of moment-generating functions to describe features of distribution. This allowed him to study how the shape of the distribution changes as random outcomes are added together. Laplace demonstrated something truly remarkable: irrespective of what is being summed, as the number of outcomes we sum increases, the moments always become closer and closer to those of the normal curve.
While there were tricky exceptions to be grappled with:
the result that Jarl Lindeberg finally proved in 1920, it is known today as the central limit theorem, or CLT. It says that whenever we add up lots of independent random measurements, each with mean and standard deviation σ, then the sum of those measurements has a bell-shaped normal distribution with a mean μ and a standard deviation of σ.
To take in the vast scope of this result, consider just a few examples. If we sum the results of 100 dice throws, they are normally distributed. If we sum the outcomes of repeated games of dice, cards, roulette wheels, or online casinos, they are normally distributed. The total scores in NBA basketball games are normally distributed (illustrated in the bottom panel of figure 3). Crop yields are normally distributed. Speed of traffic on the highway is normally distributed. Our heights, our IQs, and the outcome of personality tests are normally distributed.
Whenever random factors are added up to come, or whenever the same type of observation is repeated over and over again, the normal distribution can be found. De Moivre, Laplace, and, later, Lindeberg created the theoretical bounds within which the confidence equation can be applied. What they couldn't know, and what scientists have since found, is just how many phenomena can fit under that same curve.
A bridge to markets
We now jump to chapter 6 to see how this concept applies to investing.
Chapter 6: The Market Equation
Sumpter lays it out:
dX = h * dt + f(X) dt + σ * ε
This looks very similar to the stock diffusion equation known as Brownian motion where h is the drift and σ * ε is the random component. The signal and the noise respectively.
But there’s a wrinkle in this version.
We acknowledge:
The key assumption for the central limit theorem is that events are independent. In roulette, one spin of the wheel doesn't depend on the last; the central limit theorem applies.
But not all financial mathematicians understood that the central limit theorem didn't apply to markets.
❗This brings us to the f(X) term in the market equation. I haven’t seen that before.
It is a feedback function.
We turn back to Sumpter:
When I met J. Doyne Farmer in 2009, he told me about a colleague at one trading firm—which, unlike Farmer's own company, had lost a lot of money during the 2007/08 crisis—who referred to the Lehman Brothers investment bank crash as a "twelve sigma event." As we saw in chapter 3, 1-sigma events occur 1 time in 3, 2-sigma events occur about 1 time in 20, and a 5-sigma event about 1 time in 3.5 million. A 12-sigma event occurs 1 time in, well, I'm not sure, actually, because my calculator fails when I try to find anything larger than a 9-sigma.
The simple signal and noise market model assumes independence in price changes. Under the model, future values should thus follow the √n rule and the normal curve. In reality, they don't.
On the stock market, one trader who sells causes another to lose confidence and sell too. This invalidates de Moivre's central limit theorem. Fluctuations in share prices are no longer small and predictable. Stockholders are herd animals, following each other into one boom and bust after another. Introducing the f(X) term into the equation means that traders don't act independently from each other, but it does assume they have short memories. It again invokes the Markov assumption, this time to say that traders' feelings about the near future change as a function of their feelings now. Seen this way, the Market equation can be thought of as combining the Confidence equation, for separating signal and noise, with the Influencer equation, for measuring social interactions, in a single model.
Instead, as the theoretical physicists in Santa Fe showed, the variation in future share prices can become proportional to higher powers of n, such as n²/³ or even proportional to n itself.
This makes markets scarily volatile.
While I haven’t seen this market equation before, the topic is not foreign. In Thinking In N not T you learn how the presence of auto-correlation underestimates an asset’s volatility!
In a world where even laypeople know Taleb’s favorite gym lift, it’s banal to point out that we don’t inhabit Mediocristan. And yet, experience suggests it’s not so banal that we’ve internalized the implications of non-gaussian distributions.
Maja finds that non-mathematicians seldom take the time to reflect on the assumptions that underlie the models she uses. They see what she does as predicting the future, rather than describing future uncertainty. Last time we met for lunch, together with her colleague Peyman, she told me, "The biggest problem I see is when people take the results of models literally." Peyman agreed. "You show them a confidence interval for some time in the future, and they take that as true. Very few of them understand that our model is based on some very weak assumptions."
What’s possibly more upsetting is what the equation means for people that spout “reasons”.
The core message of the market equation is to be careful, because almost anything could happen in the future. At best, we can insure ourselves against fluctuations without needing to know why they have occurred. When the markets temporarily melted down and bounced again at the start of 2018, Manoj Narang, CEO of quantitative trading firm MANA Partners, told the business news organization Quartz that "understanding why something happened in the market is only slightly easier than understanding the meaning of life. A lot of people have educated guesses, but they don't know."
If traders, bankers, mathematicians, and economists don't understand the reasons markets move, then what makes you think that you do? What makes you think that Amazon shares have reached their peak or Facebook shares will continue to fall? What makes you so confident when you talk about getting into the housing market at the right time?
Sumpter leaves us with what I’d describe as irreducibly vague advice:
The most important lesson from the market equation, a lesson that applies not only to our economic investments but also to investments in friendships, in relationships, in work, and in our free time. Don't believe that you can reliably predict what will happen in life. Instead, make decisions that make sense to you—decisions you truly believe in. (Here you should use the judgment ie Bayes equation, of course.) Then use the three terms in the market equation to prepare yourself mentally for an uncertain future:
Remember the noise term: there will be many ups and downs that lie outside your control.
Remember the social term: don't get caught up in the hype or discouraged when the herd doesn't share your beliefs.
And remember the signal term: that the true value of your investment is there, even though you can't always see it.
Finally, here’s a fun excerpt that I want to point out because everyone knows this person — Mr. My Way:
I am sitting in a café in the late afternoon and watch him come in. He shakes a waiter's hand and then does the same thing with the barista, exchanges smiles and a few words. He doesn't see me at first, and as I stand up to go over to him, he spots someone else he knows. A round of hugging ensues. I sit back down again, waiting for him to finish.
His celebrity here partly derives from his former life as a professional soccer player, and because his face is often on TV, but he is also popular because of how he holds himself: his confidence, his friendliness, the way he takes the time to talk to people, sharing a few words with everyone.
Within a few minutes of sitting down with me, he is into his spiel. "I think I make a difference because I show them my way of doing things. I think that's lost sometimes," he says. "I just do my thing, I tell it as it is, and I am honest, because that's what is needed in this game. "I've got a lot of contacts. A lot of meetings like this one, you know, keeping connected. You see, people want to talk to me because I have unique way of seeing it. Because of my background, you know, a way that no one else has quite got, and that's what I'm aiming to de liver when I sit down with you." These observations are interspersed with anecdotes of his playing days, a bit of name-dropping, and rehearsed stories, complete with well-timed jokes.
He smiles, looks me straight in the eyes, and, at times, makes me feel like I've asked for all this information. But I haven't asked for it. I wanted to talk about using data, both as it is employed in the media and within the game of soccer. Unfortunately, I'm not getting anything useful. I call this type of man "Mr. My Way," after the song Frank Sinatra made famous. The careful steps, the standing tall, and the seeing it through provide the basis for each of his stories. It can make a beautiful melody, and for the two or three minutes during which my current Mr. My Way is hugging and greeting his way into the café, it entertains those he meets. But it only works provided he moves from one person to the next. Now, here am I, stuck in this position, with nowhere to go.
I've enjoyed hearing behind-the-scenes stories about players and big matches, and finding out about life at the training ground. Moving from being a fan to being someone who is confided in by those close to the action was, to use the biggest cliché possible, a dream come true. I still love hearing those stories and seeing the real world of my favorite sport for myself. But more often than not, the interesting bits are accompanied by "heroic" tales of Mr. My Way's "vision," followed by accounts of how their progress has been foiled by a cheating adversary or how they could do things better than anyone else if they had been given half a chance. Because of my background in math, these guys often feel they have to explain their thinking process to me. They start by telling me that I have a different way of looking at things than they do, without actually asking me how I look at things. "I think stats are great for thinking about the past," he will tell me, "but what I bring is insight into the future."
After that, he will explain how he has a unique ability to spot a competitive advantage. Or how it is his self-confidence and strong character that help him to make good decisions. Or how he has cracked a way of picking out patterns in data that I have (he assumes) missed.
His tales tend to include a digression to times that didn't go quite as well for him. "It was only when I lost concentration that I started to make mistakes," he tells me. But he always returns to emphasizing his strengths: "When I stay clear and focused, I get it right."
What I hadn't understood when I started working with sports statistics was just how much time I would have to sit listening to men telling me why they believed they were the special one. I should have known better because this doesn't just happen in sports. I have experienced the same thing in industry and business: investment bankers telling me about their unique skill sets. They don't need math because they have a feeling for their work that their quantitative traders (known as quants) can never have. Or tech leaders explaining to me that their start-up succeeded because of their unique insights and talents. Even academics do it. Failed researchers describe how their ideas were stolen by others or, when they succeed, they tell me how they stuck to their principles. Each of them did it their way. Here is a difficult question to answer: How do I know whether someone is telling me something useful or not? The guy I'm sitting with now is obviously full of it. He has talked about himself nonstop for the last hour and a half. But many other people do have something useful to say, including, on occasion, Mr. My Way. The question is how to separate the useful stuff from the self-indulgent stuff. The difference between a Mr. My Way and a mathematician can be summed up in one word: assumptions. Mr. My Way barrels through the world confident everything he assumes is true really is true.
In case you start feeling too smug about the bullshitters in your work sphere, stop to consider the second order effect of knowing that some signals are more verifiable than others. See the 1-min read The Paradox Of Provable Alpha.